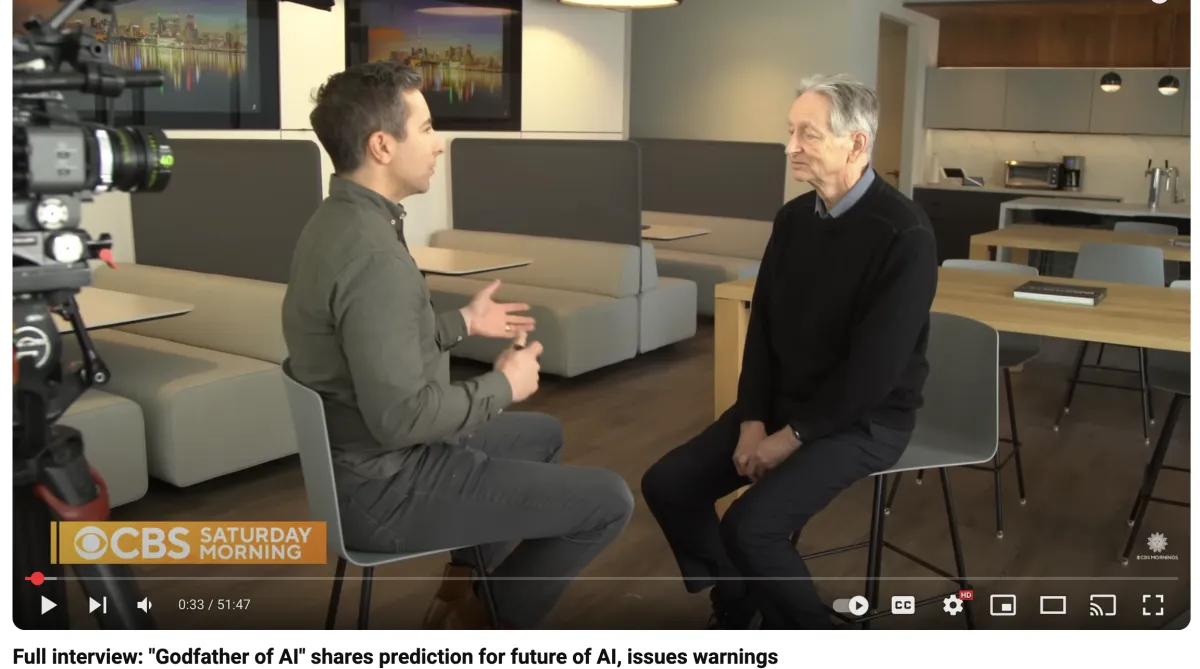
"Godfather of AI" shares prediction for future of AI, issues warnings
Geoffrey Hinton (“Godfather of AI”) tells CBS that frontier models have progressed faster—and grown riskier—than even he expected two years ago. He foresees a “good chance” we hit super-intelligence inside 4-19 years, gives a 10-20 % probability that AI agents wrest control from humanity, warns that open-sourcing model weights is “like selling fissile material on Amazon,” and calls for heavy-duty safety R & D plus government oversight before the tiger cub grows up. Yet he also outlines huge upsides—AI doctors, tutors, materials science—and thinks the public will demand regulation once it understands what’s coming.
1. Timeline & capability jump
Progress “scarier than I thought”; autonomous agents add real-world action, not mere answers.
Updated AGI clock: good chance arrives in 4-19 years, maybe ≤ 10 years.
2. Near-term benefits he expects
DomainSpecific edgeHealthcareModels will out-read radiologists after millions of X-rays; act as “family doctors” who’ve seen 100 M patients and integrate genomics + full history. Combination with a human already beats solo MDs in rare cases.Drug designFaster molecular search → better medicines.EducationPrivate-tutor AIs can diagnose misunderstandings and triple learning speed—“bad news for universities, good for people.”Climate & energyNovel materials (e.g., better batteries, maybe room-temp superconductors) plus productivity boosts across industries.Customer serviceCall-center jobs will be mostly AI, giving instant expert answers.
3. Labor impact shift
Two years ago he was relaxed; now sees large displacement for any “routine, predictable” role—call-center, paralegal, secretarial, some journalism, accounting, even many legal tasks.
Without intervention, productivity gains flow to “extremely rich”; low-income workers end up juggling three jobs.
Universal Basic Income may stop starvation but won’t solve dignity; meaningful work still matters.
4. Existential-risk numbers
Expert consensus: probability of AI takeover is “well above 1 % and well below 99 %.”
His personal guess: 10-20 % chance of loss-of-control scenario (“unfortunate agreement with Elon Musk”).
If society continues profit-driven development without safety, “they’re going to take over.”
5. Why super-intelligence tends to win
Analogy: cute tiger cub—safe today, lethal when grown if not conditioned.
Intelligence-gap argument: almost no cases where the less intelligent controls the more intelligent.
Digital agents share knowledge billions × faster than humans (parameter averaging across hardware).
6. Safety & regulation agenda
Research priority: discover if we can build systems that never want to seize power—“alignment is hard because human interests conflict with each other.”
Calls for governments to mandate:
Robust testing before deployment (praised California’s SB 1047).
Disclosure of evaluation results.
At least one-third of compute spent on safety research.
Criticizes tech lobbying for less oversight; current US administration unlikely to regulate.
Sees Anthropic as most safety-focused, but worries investor pressure will erode that.
7. Model-weights = fissile material
Releasing LLM weights slashes the cost for “small cults or cyber-criminals” to fine-tune dangerous systems.
Compares to handing out enriched uranium.
Opposes Meta’s and OpenAI’s planned weight dumps; open-source analogy is false—nobody audits trillions of floats the way coders audit open-source lines.
8. Geopolitics & arms race
Export controls on China may delay only a few years; massive domestic investment will close gaps.
Cooperation is unlikely on autonomous weapons but possible on existential-risk safeguards (like US-USSR nuclear accords).
Points out we must define which nations still qualify as “democracies” before framing “democracies vs authoritarian” race.
9. Other ethical knots
Robot rights: rejects the idea—“I eat cows because they aren’t people; AI isn’t people either.”
Embryo selection: supports choosing against severe disease risk; admits it’s complicated.
Creative-content training: analogizes AI learning from data to musicians learning genres—scale, not principle, differs; still favors protecting artists’ income streams.
10. Personal anecdotes & shifts
Left Google in 2023 after epiphany on how digital nets swap gradients across thousands of machines.
Recalls surreal 1 a.m. call telling him he’d won a Nobel Prize in physics—thought it was a dream (he’s a psychologist-turned-CS researcher).
Practical precautions: now splits savings across three banks fearing AI-powered cyber-attacks on the Canadian banking system.
11. Immediate action checklist (for policymakers & labs)
Freeze weight-sharing for frontier models; allow only controlled API access.
Legislate test-and-report duties; revoke patents or issue fines for skipped red-team checkpoints.
Allocate ≥ 33 % compute at major labs to alignment, robustness, deception-eval research.
Fund joint “AGI safety Manhattan Project” with international participation—including China—focused on provable control methods.
Educate the public: clear risk communication will unlock political will for oversight.
Key takeaways for your notebook
Timeframe: AGI plausible this decade; super-intelligence almost certainly within 20 yrs.
Risk band: ~10-20 % existential; > 50 % major job upheaval.
Leverage: Safety research, weight-control, and regulation are still possible—but window is closing fast.
Opportunity: Healthcare, tutoring, materials, productivity booms—if we keep the tiger on our side.